How do you use a search engine to find images? What keywords will you use to improve your search results?
It can be difficult to translate what we want to see in an image into words. Humans can identify thousands of intertwined visual components of an image that aren’t easily described by search terms. We can even imagine what an image might look like without knowing whether it even exists. A search engine lacks that creativity.
The power of the average human brain, at least at the moment, far outstrips the ability of any computer-based technology. While the brain is technically millions of times slower at performing basic computational steps than a computer, Liqun Luo at Stanford University reminds us that “humans triumph over computers in numerous real-world tasks—ranging from identifying a bicycle or a particular pedestrian on a crowded city street to reaching for a cup of tea and moving it smoothly to one’s lips—let alone conceptualization and creativity.”
This semantic gap, or the disparity between an image’s digital and human interpretation of image content, tends to limit accuracy and success of these searches. It’s difficult for search engines to understand the context, meaning, and objects within an image solely based on pixel information. In fact, even when it seems like you’re getting search results based on an image’s content, you’re actually getting results based on the text associated with the image, what librarians call “metadata.” If the metadata for an image is limited because there’s very little text associated with an image its digital record, or if the descriptions are subjective or inaccurate, it can make this task even more difficult.
* * *
AI-based visual search tools are already beginning to change this. If you have an iPhone, for example, you may notice that you can search simple terms like “dog” or “food” in your photos and access images that include those things with modest accuracy (when I search “dog” in my photos, I get twenty-one pictures of cats and one shoe). Amazon Lens allows you to take photos of objects in your space and search for similar products.
In the future, image recognition, object detection, and enhanced metadata extraction tools like this, which allow the computer to generate metadata from the image itself, rather than looking for human-generated text associated with the image, will likely become much more widespread and accurate as the datasets they train on expand. By combining these advancements, AI has the potential to revolutionize image search, making it easier, more intuitive, and capable of understanding and fulfilling user needs more accurately. AI promises a future where people can easily search for images with shared visual features, a task that’s nearly impossible with currently publicly available tools, as even minor variations in color, angle, lighting, or composition can make it difficult to identify similar images accurately.
At the moment, though, successfully finding images relies heavily on how well search terms connect with image metadata. Sometimes this includes descriptions of an image’s visual content, but often it doesn’t. And when it doesn’t, searches that describe visually what you’re looking for are unsuccessful. While AI tools in the future may help bridge the gap between what we expect to/want to see and how we access it, for now, understanding metadata and applying those skills is the best way to be successful locating images.
* * *
So, what is metadata? Metadata refers to descriptive information that provides details about other data, like documents, files, images, and videos, whether digital or physical. Describing metadata for novels, for example, José Calvo Tello says, “Metadata is knowledge about the text, formalized in a way that is computationally accessible.” Metadata’s ability to be computer-readable is what allows us to sort, search, and ultimately access images in databases like JSTOR.
Essentially, metadata is data about data. When you open a folder on your computer and see a list of file names with their dates and file types, you’re looking at a list of metadata! Metadata can include a wide range of information, depending on the context and purpose. When it comes to digital images, metadata typically includes:
- Descriptive metadata providing information about the image itself like a title, description, keywords, or material type.
- Administrative metadata describing the creation, ownership, and management of the image like the creator, creation date, owner, rights, and permissions.
- Technical metadata is not always visible on a user’s end but includes technical aspects of an image like file format, size, resolution, etc.
Good metadata associated with an image can fill in a lot of blanks when you’re looking to learn more about an image. This information can also bridge the gap between your search and the images you are looking for. When searching in any platform for images, the words you input are compared directly to the words in images’ metadata. Descriptive and administrative metadata (like the creator, culture, title, and work type from the JSTOR example above) tend to be the most useful for accessing images through a search, largely because these are still human-generated and reflect the language that end-users are likely to input when searching.
* * *
Searching with metadata in mind allows users to leverage additional information about images, making it easier to locate specific images, filter search results, and improve relevance and precision. When you have a clear understanding of your image need, the next step is to consider metadata and navigate any barriers that come with using text to search for images.
- Consider what metadata might be attached to your goal images. This might be as easy as identifying the name of an image you’re looking for or the name of the image’s creator. Unless you’re seeking a specific artwork, though, this may not be an option. Names of places, types of material, and dates can also return relevant search results.
- Conduct preliminary research. Even a quick Google search will help you identify potential terms to use when locating images. Who would have created images like the ones you are looking for? What organizations or institutions would host those images? What keywords or descriptors would someone have associated with those kinds of images? When would they have been created?
- Try more than one resource. Despite their breadth, popular search engines will not connect you to every image or even the most relevant images. Explore other image resources that might fit your need and search across multiple platforms.
- Search and search again. Most of us do this intuitively: when we perform a search that doesn’t go as planned, we immediately revise our search terms. Crafting multiple searches is essential to finding images, and doing so in a thoughtful way makes each search more successful than the last.
* * *
Searching for images is a journey, and iterative searching is key to successful image retrieval. The first search may not be successful, but it will guide you towards what you should or should not type into a search bar to get the images you need.
If you saw the image below, how would you describe it in a few words?
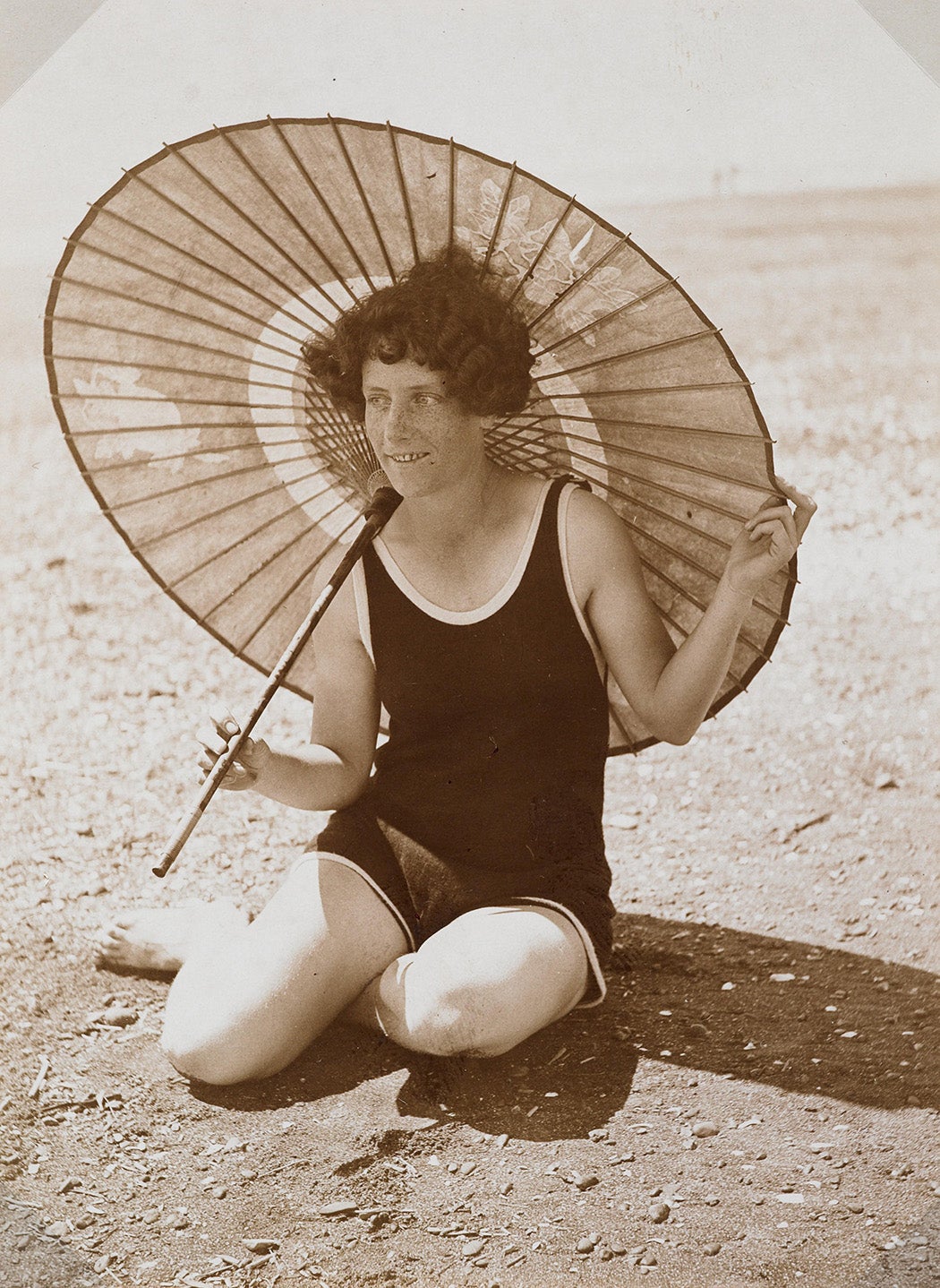
Based on the image’s metadata, if you were to search “swimming”, “umbrella”, “swimsuit”, “vacation”, “sand”, “outdoor”, or even “portrait”, this image would not come up in your search results. Sometimes different institutions or individuals generating metadata for images will use different words to mean the same thing, like dress, clothing, garment, and costume. Anticipating this and trying multiple synonymous search terms to generate different results can connect you with a wider variety of potentially relevant images. While “swimsuit” and “umbrella” would not return this image in a list of search results, “bathing suit” and “parasol” would.
The same word might also be used to refer to different things, like using “costume” to refer to clothing or to refer specifically to clothing worn during performances. When encountering unexpected or irrelevant results when searching homonyms, it helps to add clarifying keywords to your search, such as specifying “China photograph,” “china doll,” or “china plate.”
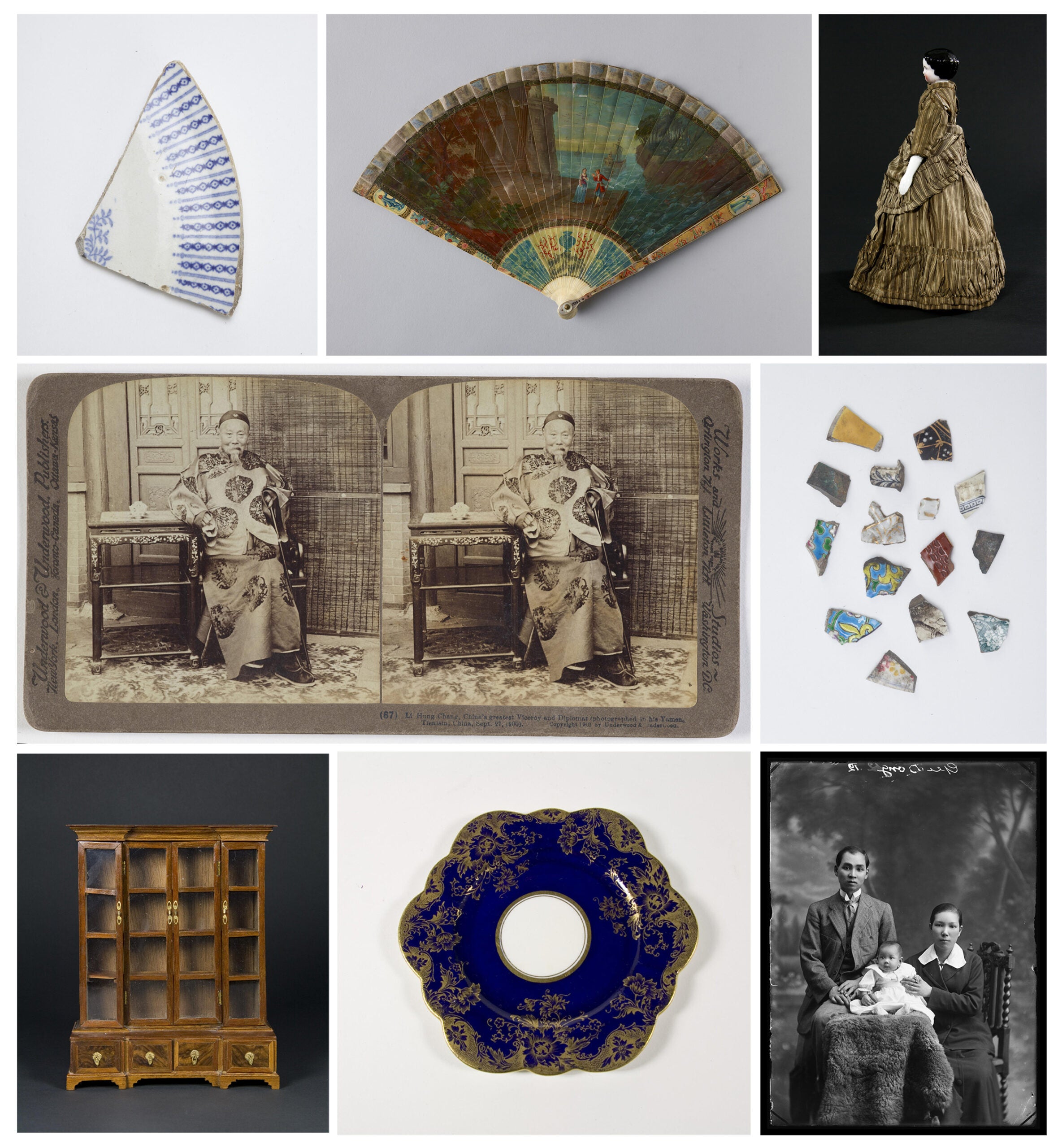
When searching in English, we must also consider that English is spoken differently and with different vocabulary across the world. Searching “bathing suit” in collections from The Metropolitan Museum of Art, an American museum, yields results you might expect if you are an American English speaker. In collections from the Museum of New Zealand – Te Papa Tongarewa, the phrase “bathing costume” is much more successful. If you were looking for swimwear from other time periods, bathing gown, tank suit, or caleçon might yield better results depending on the targeted era.
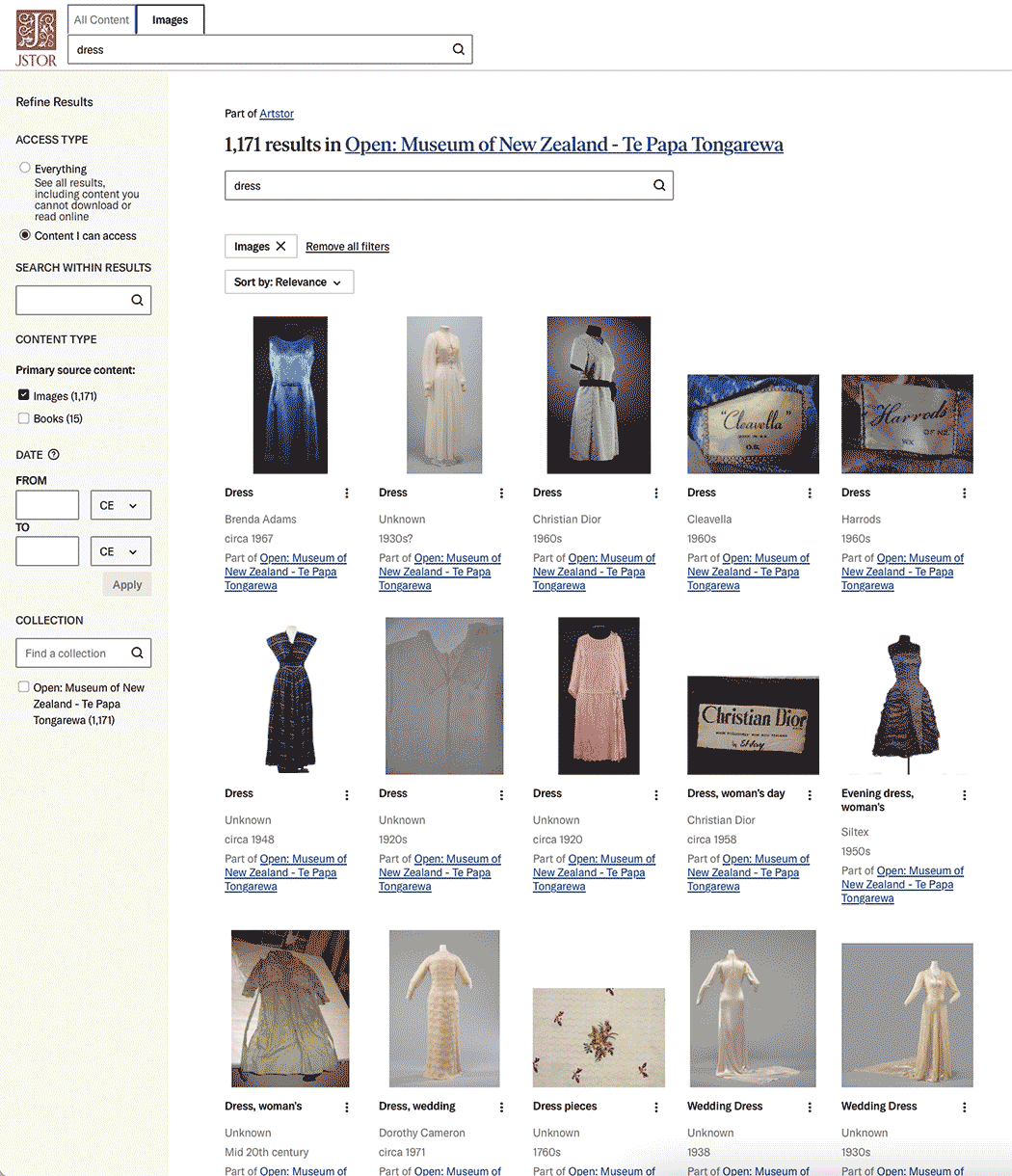
Often, an image need doesn’t translate neatly into an image title or subject, hindering easy access through a keyword search. Taking a moment to search related terms in addition to the first few that come to mind will broaden a search considerably. A search for any of the swimwear-related terms we have discussed may turn up fairly good results, but you may find that identifying common contexts for such images may yield additional, or even better, results. This could include thinking about who might wear swimsuits—bather, swimmer, surfer—where they might don swimwear—beach, pool, sand—or when a bathing suit is commonly worn—picnicking, swimming, sunbathing.
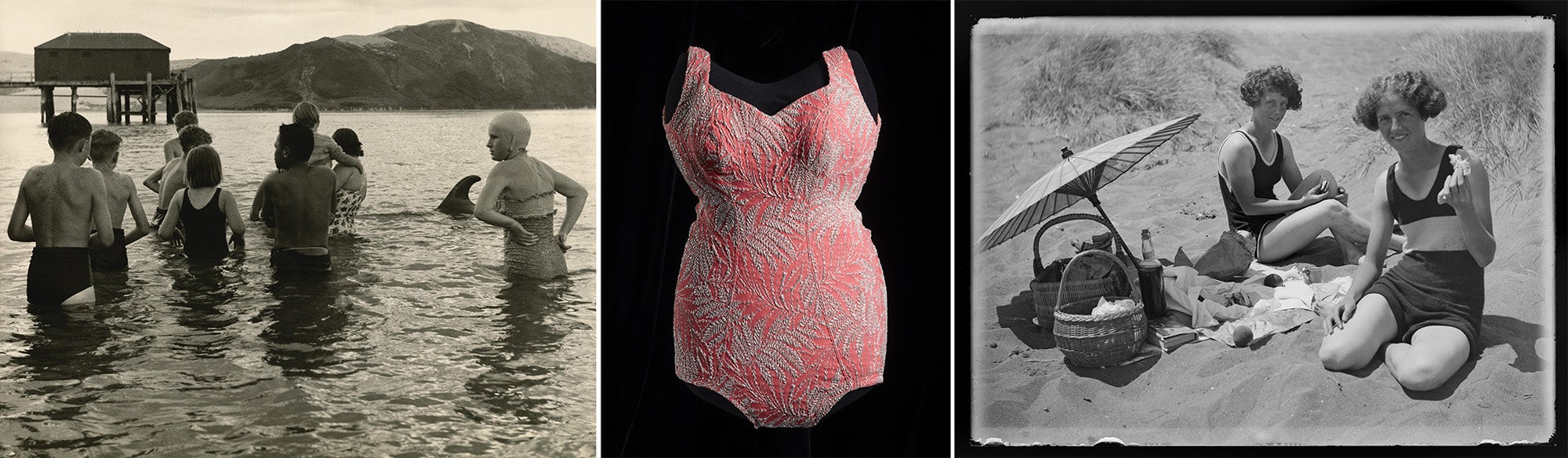
* * *
Repetitive searching is time-consuming and can be discouraging, but there are ways to streamline searches, even while expanding into related terms and other metadata fields. Two techniques are:
- Truncation: Many image searches allow you to use search truncation, or stemming. This allows you to search the root of a word with a symbol attached to the end and get results that include various word endings. Searching on swim* would return hits for swim, swims, swimming, swimmer, swimsuit, and swimwear all at once. The symbol can vary by database, but most use an asterisk, including JSTOR.
- Boolean Operators: Take a step beyond the search bar and try the advanced search feature available on JSTOR and most other image resources. The Boolean operator “OR” allows you to search multiple alternate terms at once, like swimsuit OR bathing suit OR bikini rather than performing three separate searches. “AND” placed between two terms performs a search requiring both terms to be included in an image’s metadata, like swim AND beach. “NOT” excludes results with unwanted terms in their metadata, like pool NOT geothermal.
To explore more tips for using JSTOR image search, check out our LibGuide.
* * *
Having a specific image need in mind and thoughtfully combining it with search terms that relate to available metadata can make searching for images much easier. In the next Learning to Look column, we’ll think through how to explore images more broadly and browse to encounter the unexpected.
Support JSTOR Daily! Join our new membership program on Patreon today.