A team of scientists in Austria has created a new, user-friendly artificial intelligence program to speed up their research by automating the analysis of huge numbers of plant images. They made the initial version of the source code publicly available in April 2020.
The study of plants involves identifying both their genotype (genetic makeup) and their phenotype (observable physical characteristics). Accessing the genomic sequence of an organism is a fundamental part of the study of biology. It allows researchers to make connections between a certain phenotype, such as height or color, and the genes responsible for it, says Patrick Hüther, a scientist who was then at the Gregor Mendel Institute of Molecular Plant Biology (GMI) at the Austrian Academy of Sciences in Vienna (the team is now at Ludwig-Maximilians University in Munich). Hüther is co-lead author of a research article on the development of this new artificial intelligence program, dubbed “ARADEEPOPSIS.”
Weekly Newsletter
Phenotyping plants is an essential part of agricultural, environmental, and pharmaceutical research.
With an increasing world population and the looming challenges of climate change, perfecting the science of growing food is more important than ever. Farm operators are well acquainted with influencing plant traits through a combination of genetic analysis and phenotyping in the field to produce desired characteristics in their crops. Although phenotyping is an increasing focus in agriculture, at least one study notes there’s also a growing need for large amounts of phenotype data to be processed more quickly—and in a format that researchers can easily interpret.
Likewise, understanding plants’ relationship to their habitat contributes to scientists’ knowledge about the environment. For example, one study found that the differences found in the phenotype and genotype of a plant that grows on both the coast and in interior areas do not necessarily correlate with the plant’s location. Biologists also use plants to study human diseases. Researchers have developed plant models that can predict the genes involved in human congenital diseases by comparing the plant phenotypes to phenotypes in humans and other species, identifying nonobvious similarities.
Depending on the scope of a study, collecting genotype and phenotype data can result in a mountain of information, particularly because plant development is often studied over a period of weeks or months. But, in terms of accuracy and efficiency, the technology that decodes plant DNA has far outstripped the tech that catalogs plant images. The lopsided data collection methods can result in a “phenotype bottle neck”—a backlog of thousands of images waiting to be analyzed. This bottleneck, in turn, delays researchers’ ability to analyze the data and draw conclusions.
In 2018, scientists from GMI started developing their own solution to this problem—an easy-to-use software program that could quickly process large numbers of plant images and account for color variations and other differences among plants specimens.
The name “ARADEEPOPSIS” comes from Arabidopsis Deep-Learning-Based Optimal Semantic Image Segmentation. “Arabidopsis” (Arabidopsis thaliana) is a fast-growing plant frequently used as a model organism by researchers. “Deep learning” refers to a teachable, multi-layered type of artificial intelligence inspired by the function of the human brain that spots patterns and interprets data.
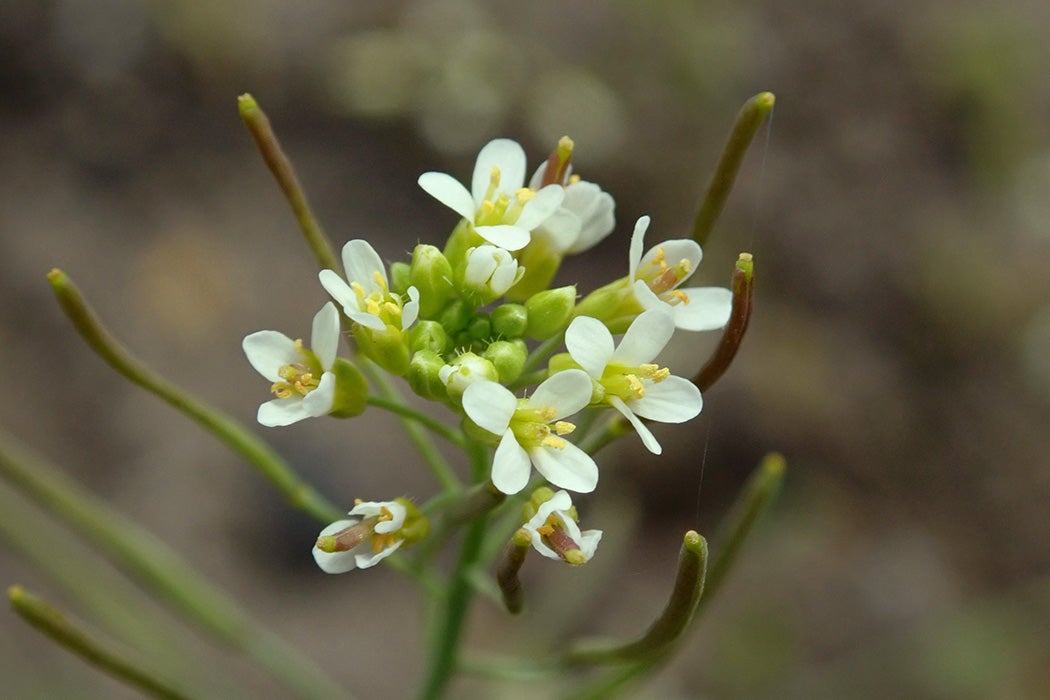
The impetus initially came from GMI researcher Niklas Schandry’s own phenotype bottleneck, when he found himself faced with 150,000 plant images to analyze as part of a study to understand how different types of soil affect the way plants grow. Existing image analysis programs could quickly process the images but could only identify the plants’ green areas. This limitation was a problem since Schandry’s research found that certain soil types caused plants to turn yellow and brown, he explains.
Going through thousands of images to identify even a small set of plant characteristics could easily take a botanist weeks or months. “It’s a very dull task and hard to do reliably,” Schandry notes.
Then Hüther, Schandry’s fellow scientist at GMI, happened to read a Google AI blog post on semantic image segmentation, which assigns a descriptive label to every pixel in an image. Fortunately, Google made the semantic image segmentation model publicly available, so Hüther started playing around with the code. Ultimately, he repurposed the code for plant phenotyping by teaching the software how to identify Arabidopsis specimens. “There was a lot of trial and error at first, but eventually I figured out how to turn it into an end-to-end pipeline that also other researchers can use to analyze their images,” says Hüther.
Using deep learning methodology, ARADEEPOPSIS accurately analyzes Arabidopsis rosettes—the plant’s circular arrangement of leaves as seen when viewed from overhead—regardless of the plant’s color variations. Importantly for many botanists’ work, ARADEEPOPSIS can reliably distinguish between healthy and unhealthy leaves. The program also takes into account variations in plant appearance, image quality, and background composition.
So, how much time and effort can ARADEEPOPSIS save researchers? Quite a bit.
Hüther estimates that, depending on the computer hosting it, ARADEEPOPSIS can analyze 100,000 images in one day, which includes extracting a total of 78 phenotype-related parameters from each image. If an individual takes 10 minutes to identify 78 phenotypic parameters for a single image, that person will need to work 40 hours a week for approximately eight years to complete the analysis of 100,000 images, he says.
Not that any researchers in their right minds would take on such a workload. Programs to automate phenotyping for large numbers of plant images already do exist, including PlantCV, open-source software developed by the Donald Danforth Plant Science Center in St. Louis. However, PlantCV requires users to have some computer programming expertise, points out Hüther.
“One of our main goals really was to build something that was very accessible and easy to use and we thus focused on full automation, something that the machine learning methodology enabled us to achieve,” he says. ARADEEPOPSIS “merely requires input images of plants and returns a rather huge table with measurements along with a visual presentation of the result, allowing for quick and easy quality control.”
Hüther, Schandry, two GMI colleagues, and a colleague from the Max Planck Institute of Developmental Biology in Tübingen, Germany, published an article about developing ARADEEPOPSIS in the scientific journal The Plant Cell in December 2020. They made the first version of the source code publicly available on Github in early 2020.
Currently, ARADEEPOPSIS is configured to analyze Arabidopsis plants and other members of the same plant family, but the machine learning program can be trained to analyze other types of plants and adapted to other researchers’ needs, says Schandry. Training ARADEEPOPSIS proved to be a very time-consuming task that included teaching the machine learning program to differentiate between “green,” “not green” and “partially green,” he says.
Potential future applications of ARADEEPOPSIS could be wide-ranging, according to Anne C. Rea, assistant features editor at The Plant Cell. ARADEEPOPSIS is customizable and more accurate than existing tools. It is also highly versatile because it can handle extremely large numbers of diverse images of varying quality and background compositions and performs a wide variety of different types of measurements, Rea writes in her overview of ARADEEPOPSIS for the journal.
Schandry and Hüther and are also looking at future possibilities. Schandry says he hopes to develop a mobile version of ARADEEPOPSIS, which would be very useful in the field for botanists.
“I am very eager to see where this will lead to, hopefully beyond the model plant Arabidopsis thaliana,” adds Hüther. “Even if that means we have to find a new name for the software.”
Support JSTOR Daily! Join our new membership program on Patreon today.